Introduction
The food sector has seen a transformation in the twenty-first century, driven by the rapid advancement and acceptance of digital tools. From farm to fork, every stage of the food supply chain is being redesigned and optimized using technology. Moreover, digital tools, encompassing a broad spectrum of technologies such as Artificial Intelligence (AI), the Internet of Things (IoT), and blockchain, are revolutionizing the food chain. In 2023, FAO, in collaboration with Wageningen Food Safety Research (WFSR) published a technical background document to raise awareness of predictive early warning tools for the prevention of food safety hazards. This summary article aims to report the main findings of the report, and support its stated objectives:
- Support awareness of early warning tools and systems for emerging issues in food safety.
- Promote research on the applications of Big Data and AI in food safety early warning systems and emerging risk identification methods.
- Provide an overview of available early warning tools and consider solutions for addressing gaps in their implementation.
- Provide practical examples of open-access tools to support food safety early warning and identification of emerging issues.
Current methods and systems for the identification of emerging issues in food safety
FAO's 2022-2031 food safety priorities emphasize the importance of food safety in support of food security and nutrition, as well as the negative impact of unsafe food on people, economies, and the environment. Food safety is essential for achieving food security. Early warning systems can reduce the risk of hazards occurring and provide timely warnings. Identifying dangers early can prevent them from becoming a serious risk. Furthermore, they can also prevent the spread or recurrence of an existing threat.
A range of different approaches and systems can be used for the timely prediction and identification of food hazards and their risks to food safety and differ in the time frame in which they operate—present, near, or far future as shown in Figure 1. Examples are discussed in the sub-chapters.
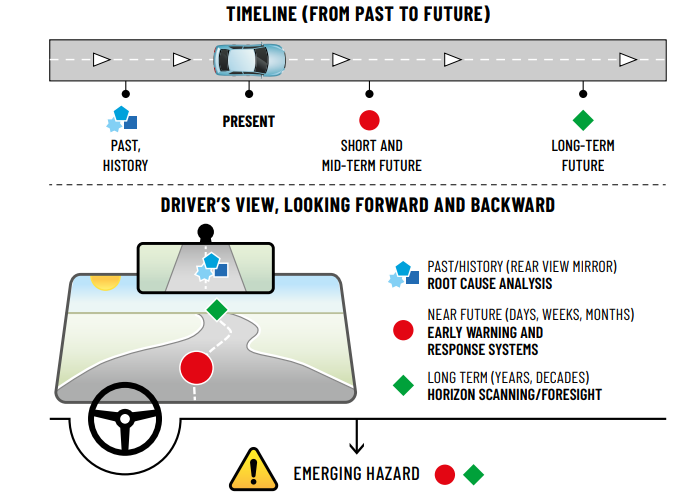
Immediate and short-term: early warning and response systems (EWARSs)
EWARSs and associated risk management procedures can aid authorities in taking necessary measures and relying on expert interpretation and prioritization of hazardous input data. Time is a vital component in early warning systems as some food safety issues have limited warning time, making it difficult to take preventive steps. Three categories of EWARSs systems exist in food safety:
Risk-based predictive systems: Based on current knowledge of potential hazards, which is fed into predictive modeling systems. Methods for the prediction of contaminants like mycotoxins are an example.
Reactive food safety-hazard focused EWARSs: Management of already identified presence of hazards in food. Rapid alert systems like RASSF are an example.
Reactive foodborne illness (“event”)-focused EWARSs: Prevention of further occurrence and spread of already reported foodborne diseases and poisoning. Input information may come from traditional disease surveillance or open media sources.
Medium-and long-term warning systems: Foresight
To provide governments with decision-making, the foresight method involves gathering facts, exploring future situations, and creating a vision to identify policy solutions. Furthermore, it also helps allocate resources and establish solutions to address future concerns.
To learn more about foresight, read this summary article about FAO's foresight report of 2023.
Big Data and AI applications in food safety early warning and emerging risk identification
The World Health Organization (WHO) defines “Big Data” as “the emerging use of rapidly collected complex data in such unprecedented quantities that terabytes, petabytes, or even zettabytes of storage may be required”.
- In recent years, using “Big Data” to address food safety concerns is becoming increasingly common.
- Food safety data can be gathered from various sources- the Internet of Things (IoT) and radiofrequency identification (RFID), in addition to traditional methods.
- Data can be processed and analyzed further by early warning and emerging risk identification tools.
Examples of Big Data applications in food safety
- Biosensors, used at various stages of the supply chain, may detect anomalies such as cattle activity and blood glucose levels while monitoring routine parameters such as body temperature. When connected to smartphones for convenient data access, they can enable improved data interpretation.
- IoT has been employed in a variety of applications, including beef cold chain logistics, where RFID sensors were combined with humidity sensors to allow real-time tracking. Furthermore, Blockchain expands the options for obtaining safe information by making it hard to change or tamper with data after it has been placed into the system.
Examples of AI applications in food safety
Artificial Intelligence (AI) combines computer science and datasets to enable problem-solving. This concept can also be applied to food safety, as offered by the following real-life examples:
- Bayesian networks (BNs), a common machine learning method for structured data processing, have proven useful in food safety. For instance, BNs have accurately predicted food fraud categories (80%) and chemical hazards in products from three countries (95%).
- Neural networks (NNs), analyzing unstructured data, have been used in various applications. For instance, in a model to identify illegal stimulants in food supplements.
Gaps and barriers to the uptake of digital tools
Big Data and AI enhance early warning tools, but their deployment, especially in LMICs faces several challenges. Some of the gaps and barriers to digital technologies applicable to food safety are:
- Lack of facilitations for sharing of information between different countries
- Lack of wide expertise, especially in AI and IoT, and long training time
- Issues with transparency and interpretability of data
- Lack of data quality and quantity in the “food safety domain”
- Challenges with scalability, data storage, data integrity
- Challenges in data fairness i.e. Findability, Accessibility, Interoperability, and Reusability (FAIR)
- High investment costs and large resources needed
- Lack of standards, for instance with communication protocols for IoT devices
To read the full list of gaps and barriers, read the full article here.
Prospects and innovative solutions
The widespread implementation of current food safety equipment involves technological and social challenges. For instance, proper infrastructure for data collection, storage, and processing is critical, particularly in low- and middle-income countries. Furthermore, socioeconomically, better coordination of food safety initiatives, stakeholder collaborations, and capacity building through extensive training are critical.
The real-life case of the implementation of AI in healthcare has already brought up a variety of ethical and policy challenges. Examples are privacy issues with personal data, discrimination against certain groups under-represented during the training stage, and wrongful influence on human judgment by AI outcomes. Addressing difficulties with AI applications requires ensuring that they are legal, ethical, and robust. Furthermore, trustworthy AI should follow values such as accountability, transparency, fairness, dependability, safety, privacy, security, and inclusivity. The complexity of AI algorithms and data quality may result in unpredictable outputs, demanding additional research on AI.
Open access tools to support food safety
To promote uptake from the food industry of digital technologies for food safety, this chapter provides practical examples of open-access tools to support food safety early warning and identification of emerging issues.
The Medical Information System (MedISys): MedISys is a 24/7 media monitoring system that assists professionals (e.g. authorities) in conducting incident-based surveillance by monitoring reports of incidents in the media. MedISys collects and analyses information provided by Europe Media Monitor (EMM) based on automatically pre-defined preferences, users can further organize articles and create newsletters to share with their target audience
MedISys-FF: MedISys-FF, a food fraud-focused dashboard based on a MedISys filter, presents information on relevant articles, their origin countries, top keywords, and publication dates. It updates every 10 minutes, 24/7, and is free to use.
SGS DIGICOMPLY: SGS DIGICOMPLY provides preventive solutions for addressing food safety hazards by compiling data on regulations, supply chains, testing, media, trading, and environmental factors. It has collected over 5 million papers from various sources, including food safety authorities, scientific publications, regulations, legislation, and social media.
IMIS Food Real-Time Food Assurance
Like the platform mentioned above, iMIS Food is a knowledge-based software helping companies to comply with food safety, regulations and standards. The platform incorporates the Deming circle for continuous improvement (Plan, Do, Check, Act) and features a compliance portal for the timely identification of food safety issues. This comprehensive approach to real-time food assurance prepares food companies for the future.
iMIS Food offers:
- The knowledge, content, infrastructure, systems, and real-time updates for Food Safety Compliance;
- Updates on legislation and food fraud cases;
- Compliance with EU legislation and standards;
- Direct access to our Food Safety experts;
- The system also works offline;
- Dutch, English, German, and French content.
iMIS Food platform for food safety
Furthermore, the website offers a free access overview of food safety information. The overviews include purchase specifications, pathogens knowledge sheets, HACCP manuals, etc.
Information overview of QAssurance
Source
FAO. 2023. Early warning tools and systems for emerging issues in food safety – Technical background. Rome. https://doi.org/10.4060/cc9162en
Related articles to Early warning food safety AI tools
Many customers and visitors to this page 'Early warning food safety AI tools' also viewed the articles and manuals listed below: